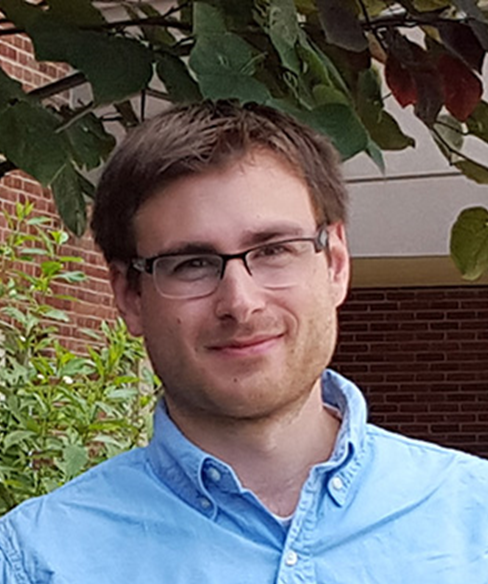
"The application of machine learning to metalloprotein engineering"
Metal cofactors perform a wide variety of essential functions within proteins. Consequently, researchers have been exploring a range of methods to rationally design structures, functions, and properties of metal sites in proteins. A number of computational tools exist that have been developed to identify sequences or structures favorable for metal binding, but their methodology has not been amenable to metalloprotein engineering. Our lab is working to develop a tool that uses machine learning to guide the design of metal sites with unique structures or desirable functions. In our efforts, we have also explored the use of machine learning in the structural classification of metal-binding sites, linking structure to functional relevance. Most of our metalloprotein engineering targets using this methodology are c-type hemes, characterized by covalent attachment to the protein via thioether bonds to a CXXCH motif. Our lab takes advantage of the versatility of heme in native proteins, the stability of the heme c conferred by the covalent attachment to a CXXCH motif, and the broader catalytic potential that can be realized upon metal-substitution of the porphyrin iron.